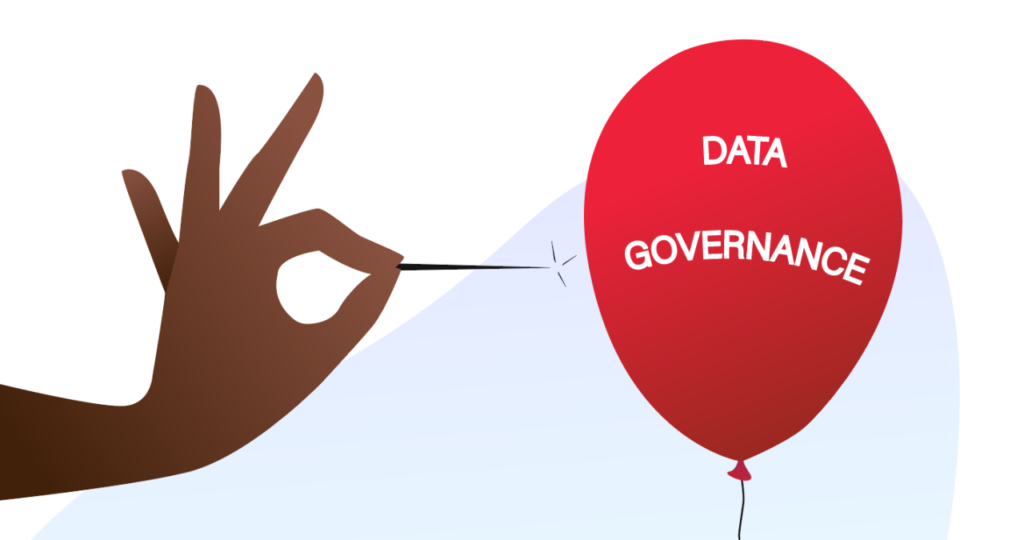
Three BIG Data Myths
Ignoring three data myths will result in the failure of your data strategy.
Myth One – All data is equal
This myth assumes that it doesn’t matter the data source, the quality of the data, or the effort it takes to make the data usable. Data sources and the value of that data need to be prioritized according to the organization’s strategic goals. If the credit union has a strategic goal to improve member access and utilization of the digital channels, the data that measures adoption, use, and the efficiency and effectiveness of the processes supporting those channels have a higher level of importance. Know what questions you want the data to answer and what strategic goals you want the data to measure will help your credit union prioritized their data needs. It is not uncommon for a credit union to have 30 or more third party data sources outside of their core system. The aggregation, normalization, and storing these data sources takes time and often money. Know which data comes first is necessary.
Myth Two – We can trust the data source
Three things impact data quality:
- The timing of the report – The ability to balance data to other sources or other reports is dependent on when the source of the data was updated and when the report was run. Some systems are updated real-time, and some are updated according to a scheduled process; on a daily, weekly or even monthly basis. The impact of this variable will cause report inconsistencies. Time stamping the report according to when the report was run compared to other report systems will help the organization to understand the report variances.
- The query used to generate the report – It is not uncommon to find different logic being used when a report is generated. These logic variables will result in result variances. To manage this, footnoting the report with the query logic will help clear up any report variables. In building a query, terms like “greater than, less than, equal to” and inclusions and exclusions in the query will help to bring clarity to any report variances.
- Definitions of report elements – How a commonly used term is defined will adversely impact the consistency and quality of the report. An example of this is a report on the number of members. If one report uses the definition, a member is a unique social security number, and another report defines a member as a unique social security number excluding members that don’t have at least $5 in a membership account; the reports will give the organization two different numbers
Myth Three – Managing data by organizational silo is the best method
We can all agree that data is an organizational asset, but we must also understand that data can be manipulated and this manipulation needs to be a. Without centralizing data quality, query controls, and definitions, the risk of manipulation of data to tell the story the report writer wants to tell versus telling the real story.
Don’t get trapped into ignoring any of these three myths as you develop your data strategy; it can be the difference between success and failure to achieve one source of truth for the organization.
To research a virtual Data Governance Course, follow this link: https://leading2leadership.com/product/data-governance-a-professional-development-workshop/